Identity is a big topic in data-driven marketing. Advertisers want to target consumers on a personal level, publishers want to monetize their audiences, and brands are trying to reach “John Smith” and TikTok customers who are currently shopping on Amazon. Identity is important to ensure that “John Smith” is scrolling through. Or the person searching for weekend events on Ticketmaster is certainly the same person.
Until now, at least across open digital platforms, mobile advertising IDs (MAIDs) and third-party cookies have been used to connect the dots. But their time is coming to an end, and the transition continues to be hectic. What options do marketers have today?
Most marketers are betting on their data because it allows them to stand out from their competitors, maintain control over their data assets, and avoid privacy issues. However, everyone uses different identifiers. Netflix recognizes you by your email address, Macy's by your phone number, Delta by your SkyMiles number, Instagram by your handle, and Xbox by your gamertag. Websites you visit without logging in may still assign third-party cookies or collect your IP address.
For consistent, comprehensive, and comparable audience measurement across platforms, marketers need a robust identity system with an identity graph designed specifically for measurement.
What is an identity graph?
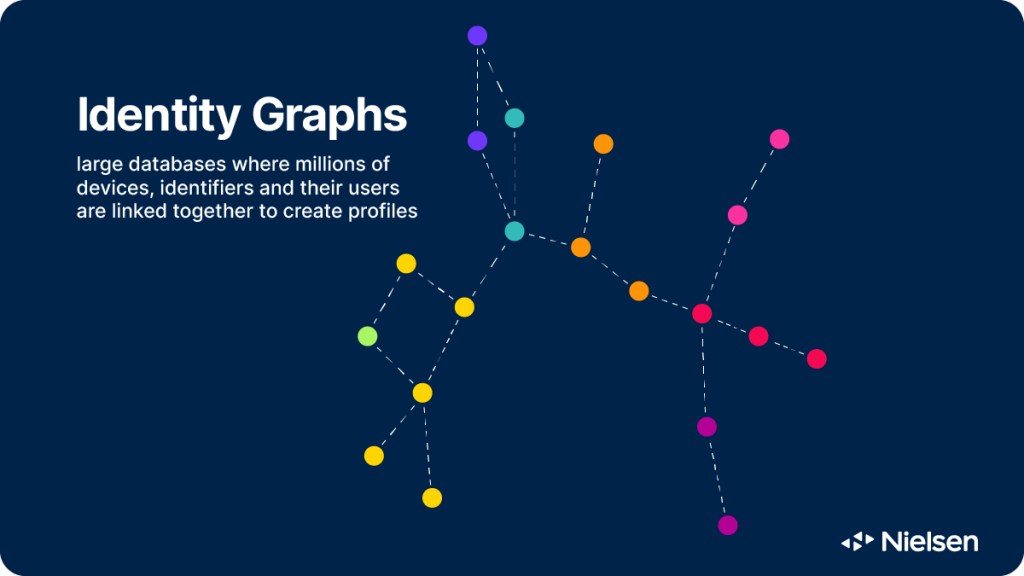
Consumers use many devices, apps, and identifiers to interact with the world, and those interactions leave a steady imprint, like snapshots in a photomosaic. Each snapshot tells just a part of the story, but when combined they tell us who we are, our likes and dislikes, our interests and preferences, and most importantly for marketers, what we can do next. A 360-degree portrait of who you are will be generated.
To bring this together, major advertisers, publishers, and data providers have developed the Identity Graph. This is a large database where millions of devices, identifiers, and their users are linked to create a unified customer and household profile. They use these graphs for targeting and personalization, matching customers and prospects on a brand's email list with audience members on the platform. For example, on the activation side of Nielsen's business, there is a graph focused on targeting.
Campaign activation is not the only reason to use ID graphs. Let's take a look at why reliable, independent, and well-calibrated identity graphs are essential for modern measurements.
How is it different from the measurement grade identity graph?
Not all identity graphs are focused on accurately representing the entire population. The data sources they use may be biased towards users in certain regions, on certain platforms, or on only one type of device. Deduplicating records may not be a top priority either, as long as some matching is done to get the ads ultimately in front of people.
However, match rate is not everything. If measurement is your end goal, statistical representation and deduplication are required to properly measure reach, frequency, and other important campaign KPIs such as return on ad spend (ROAS) and lead conversion. When Nielsen loads new data into the Identity Graph, we take great care to verify matches between devices, people, and households against census data and our own people-based panels. As we learned earlier, need to know In the series, a carefully curated, people-based panel is essential to big data coordination.
How extensive is our data cleanup process? Each year, our systems ingest billions of identifiers and links from various external data sources, but only 20 are reflected in the resolved identity graph. % only. We use People Panels to reconcile these big data sets to ensure accuracy in audience assignment and graph clustering. As a result, the Nielsen identity graph is optimized for representativeness and precision required for measurements.
How are marketers using Nielsen ID graphs?
Identity graphs are not built standalone. The Nielsen ID Graph is at the heart of a comprehensive ID system that includes four distinct steps:
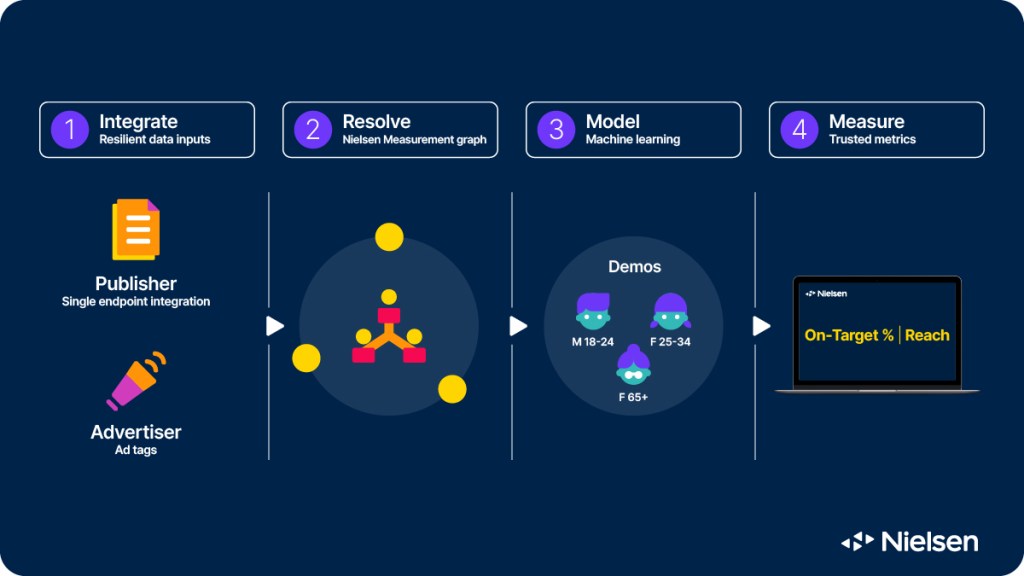
Ingest data
It all starts with capturing data relevant to your client's campaigns. This can be first-party advertiser and publisher data. Third-party data to enrich customer profiles with new attributes. Data to add volumetric insights from digital platforms. Big data such as viewing data from program distributors and smart TV manufacturers.
ID resolution
The second step is to match the new data input to the Nielsen ID graph to draw the correct links between devices, identifiers, and people. Once the data associated with each profile is verified, a unique ID (called a Nielsen ID) can be assigned to the record.
Audience and user journey modeling
The next step in the process is to compare demographics from external data sources with verified demographics from the panel to address data gaps and reconcile discrepancies. We then use advanced machine learning techniques to eliminate audience duplication and build a user journey that faithfully considers all relevant touchpoints and outcomes related to your campaign.
Campaign measurement
Finally, create audience metrics (reach, frequency, goal completion, cross-media metrics, etc.) and performance metrics (sales, ROAS, cost per lead, etc.) to understand the true, unbiased, and unduplicated nature of your campaigns. , create appropriate reports. Attributable results.
As mentioned earlier in the article, Nielsen Marketing Cloud, the activation side of Nielsen's business, uses many of the same sources as Nielsen's measurement graphs and shares the same resolution logic to provide a deduplicated view. There is an individual-level activation graph. audience. But rather than measurement, it's designed to drive campaign reach and personalization at scale.
What are the privacy implications of digital identity systems?
Ingesting ad exposure and results data from external sources can be a challenge in today's data privacy environment. Even when user consent is secured, marketers are understandably nervous about testing the limits of that consent by sharing customer data with external partners. This is especially true in highly regulated industries such as healthcare and financial services. Hashing algorithms are useful for hiding sensitive identifiers such as email addresses, but hashed IDs are considered personally identifiable information (PII) in some jurisdictions (e.g. Europe) and may not be used anyway. , it is not always possible to prevent a malicious party from identifying the individual behind the hashed ID.
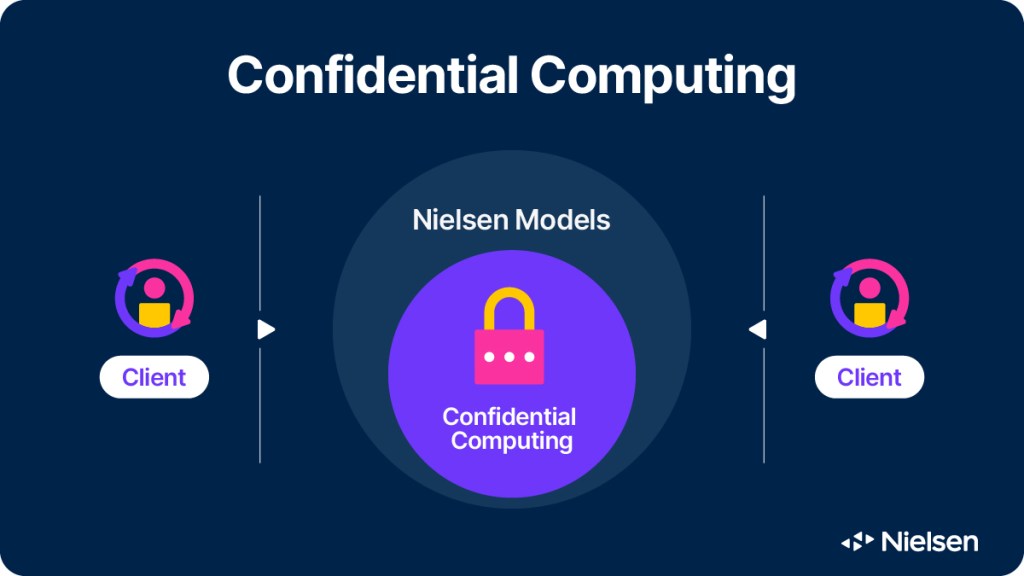
What's the solution? At Nielsen, we have a suite of data privacy and security solutions that facilitate data collaboration (such as finding overlaps between advertiser and publisher datasets) without actually sharing sensitive information between data partners. I have implemented the process. For example, cleanroom integration ensures that data from Nielsen, clients, and partners stays within their environments and can be measured.techniques like confidential computing and differential privacy Enable parties to collaborate without seeing each other's data.
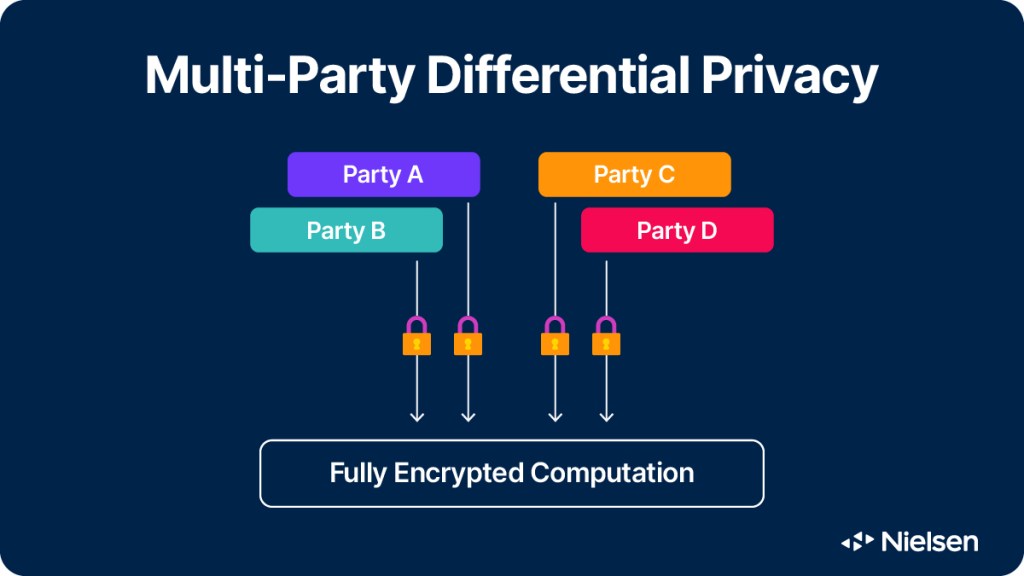
Privacy is a top priority for marketers and consumers. This needs to be taken seriously when dealing with digital identity systems.
Digital measurement beyond third-party cookies
Third-party cookies may be at the end of their game, but marketers still need to understand cross-platform campaigns. And they want to take advantage of new planning and targeting opportunities, such as those opened up by advanced audiences, to stay ahead of their competitors.
Perhaps now more than ever, marketers need a measurement partner who can connect the dots and produce accurate and consistent audience and outcome metrics that are relevant to their business.
Nielsen's need to know We revisit the fundamentals of audience measurement and demystify the media industry's hottest topics.Read all articles here.